Artificial Intelligence in Construction Quality Control
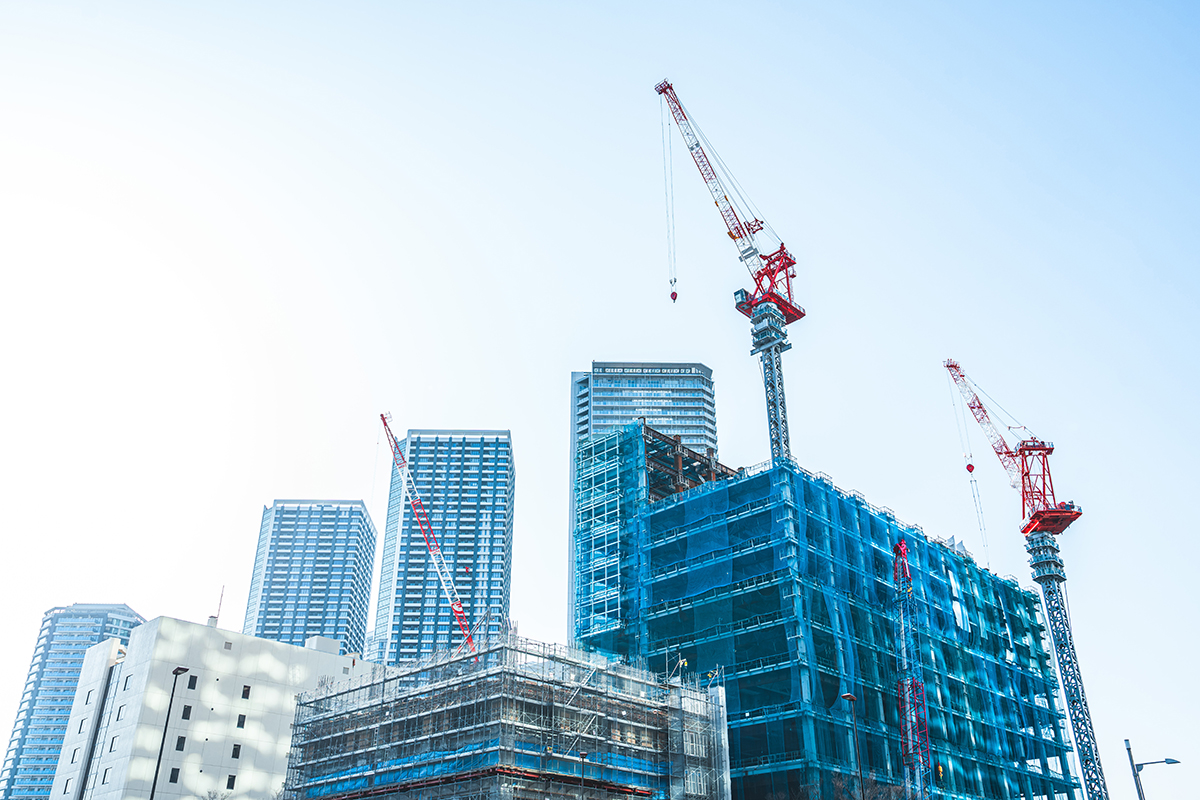
Artificial intelligence has made large inroads into various aspects of construction management, but has seemingly made less progress with on-site quality assurance and control measures. What research is being conducted on finding non-conformities or construction defects in real-time to avoid expensive, protected litigation after final completion? We take a deep dive.
Reinforcing steel does not scream tech or AI, yet it has received a considerable amount of attention in recent research. To understand why, consider some of the factors and issues concerning installation of reinforcing steel. Steel bars are delivered to a project site, placed or tied into mats by manual labor, with multiple layers of mats used in a variety of structures. Inspection of the installed rebar is performed manually. The spacing between every rebar in every mat is not measured or checked; instead, the inspector relies on a representative sample. Accessibility, particularly where multiple mats are lined up, can further hamper inspection of deeper mats. Finally, not catching non-compliant rebar spacing can lead to structures with less capacity than designed or required under applicable code, and increased cracking. Once the concrete is placed, the cost of addressing rebar spaced too far apart can be very time and cost intensive. In all of these ways, rebar presents a quality control headache with potentially drastic impact.
In an October 2021 article published in Automation in Construction, Yurii Kardovskyi and Sungwoo Moon proposed an Artificial Intelligence Quality Inspection Model (AI-QIM) built using the Mask Region-based Convolutional Neural Network (Mask R-CNN) technique with integrated stereo vision. The authors established that the technique can be used to accurately detect the spacing of rebar with 3% maximum relative error and the length of rebar with 8% maximum relative error. While this technique yielded impressive quality control results, it did not address the issue of detecting spacing and length when multiple mats of rebar are installed.
In 2024, at the 41st International Symposium on Automation and Robotics in Construction, Singyue Wang, Lu Deng and others presented on using vision based deep learning with red-green-blue-depth (RGBD) camera to determine the spacing and length of rebar when multiple rebar mats are installed. As a taste of the complexity of trying to automate and accurately model a two layer reinforcing mat, the authors provided the following background on the technique used to differentiate steel on the first layer from steel on the second layer.
Obtaining the rebar pixels is crucial for spacing inspection. However, because of the feature similarity existing in double-layer bidirectional rebar, the background rebar layer could greatly influence the inspection of rebar spacing in the top layer. To extract the pixels belonging to the top layer, this paper employed a point cloud plane fitting technique based on depth information. Firstly, the RGB and depth images, captured by using the RGBD camera, are transformed into point clouds by incorporating the camera intrinsics, and then the passthrough filter is applied to denoise the point clouds, retaining the region of interest containing the rebar points. Subsequently, the denoised point clouds undergo RANSAC plane fitting algorithm…
The technique resulted in an average error of rebar spacing inspection of 2.65mm, or 0.10 inch.
And yet, with these fairly amazing error tolerances, even the most advanced AI system still has limitations. For instance, with the deep learning model discussed above, the rebar pixel segmentation based on depth information performs poorly in capturing small-diameter rebar. In addition, other factors such as ambient noise and lighting variations introduce interference in the depth image.
While, thankfully, there will be no test at the end of this article about AI techniques to determine rebar spacing, there are some important lessons. The standard of care for construction contractors, design professionals, and third-party consultants providing on-site reviews or inspections is changing rapidly. AI is providing new techniques that allow construction to very tight tolerances and the ability to capture non-conformities or defective work in real-time, allowing corrections to be performed with minimal cost or time impact. Design professionals, typically at the leading edge of technology in the construction industry, may expect greater AI-enhanced construction quality control from construction contractors and stricter adherence to dimensions called out on the contract documents. While inspections performed by real-life humans will likely never go away, technology will quickly and increasingly play an important part in the construction quality assurance and control processes.
The attorneys in our Austin and Dallas offices routinely advise clients on the quality control and assurance processes for constructed projects. Please contact us at info@gstexlaw.com with any questions you may have.
Legal Disclaimers
This blog is made available by Gerstle Snelson, LLP for educational purposes and to provide general information about the law, only. Neither this document nor the information contained in it is intended to constitute legal advice on any specific matter or of a general nature. Use of the blog does not create an attorney-client relationship with Gerstle Snelson, LLP where one does not already exist with the firm. This blog should not be used as a substitute for competent legal advice from a licensed attorney.
©Gerstle Snelson, LLP 2024. All rights reserved. Any unauthorized reprint or use of this material is prohibited. No part of this blog may be reproduced or transmitted in any form or by any means, electronic or mechanical, including photocopying, recording, or by any information storage or retrieval system without the express written permission of Gerstle Snelson, LLP.